What impact is AI having on data centers and sustainability?
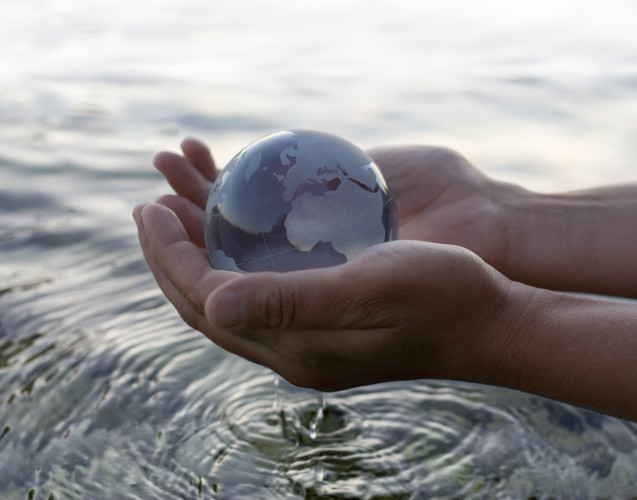
The ‘boom’ of artificial intelligence means an increase in the needs for computing and data transmission. What consequences can it have?
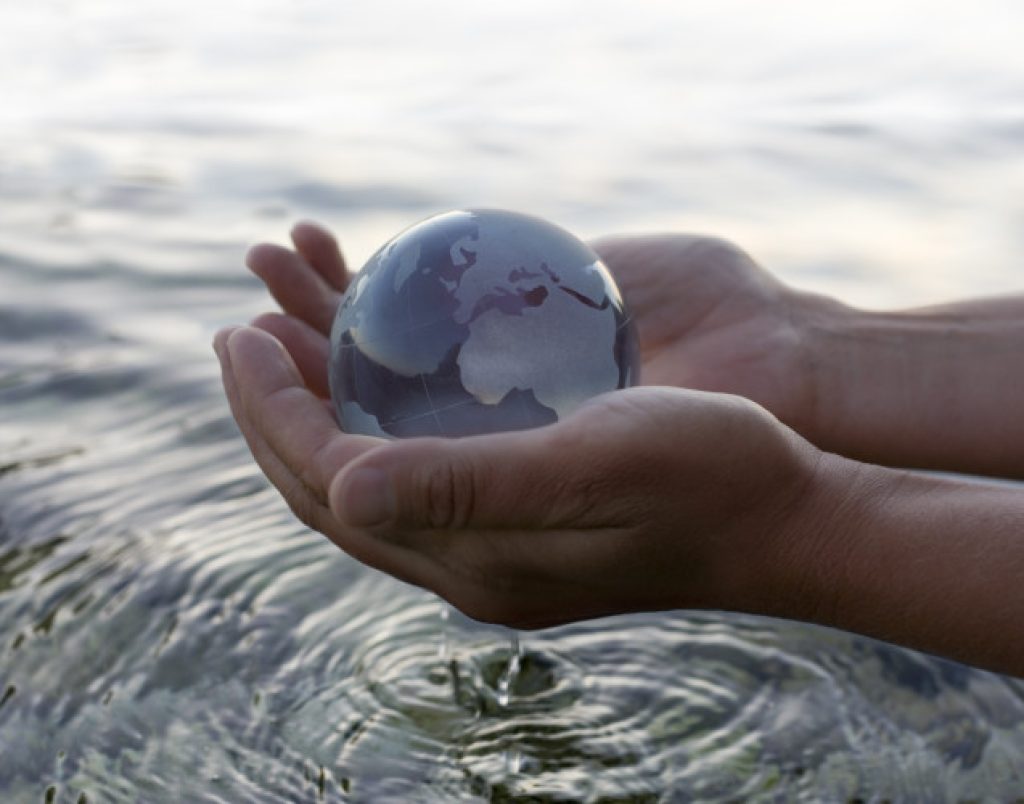
The emergence of generative artificial intelligence (AI) has given a lot of talk in recent months.
The tools supported by this technology are on everyone’s lips and we find news such as the ban on ChaptGPT in Italy, the investigation of this platform by the Spanish Agency for Data Protection or the request of Elon Musk and other businessmen and experts. of the technology sector to pause the development of AI for 6 months to analyze its risks and rethink where we want to go.
Another derivative of the development of AI is the increase in the needs for computing capacity and data transmission. “ With the development of AI, the volume of data used has multiplied exponentially . The ability to obtain results in real time is increasingly important, so it is vital to have an infrastructure network with sufficient capillarity to send, receive and process this data close to the place of emission and with minimal latency”, Ramón declares . Cano, director of Managed Services at Equinix in Spain.
Thus, Matías Sosa, product marketing manager at OVHcloud, considers that “we are facing a situation that poses three main challenges.” “First of all, we must take into account that the speed and latency of the Internet connection are crucial factors in the transmission of large amounts of data. However, the network infrastructure is not yet sufficiently developed in many parts of the world to meet all the data transmission demands that artificial intelligence entails,” he explains.
Second, he notes that “the hardware needed for AI , such as high-performance graphics processors, is relatively expensive today, and its demand continues to rise.” In addition, he reports “a possible shortage of supply could become an added problem.”
Finally, it indicates that ” AI energy consumption poses a significant challenge for our societies.” “As the demand for computing capacity continues to increase, the need for power will also increase, which will not only have implications at the cost level, but also in terms of environmental impact.”
Likewise, Federico Vadillo, an Akamai security expert , believes that “the growing demand for computing resources and the complexity of AI applications can lead to an increase in infrastructure and energy costs .” In addition, he acknowledges that “the increase in the amount of data that must be transmitted can lead to network congestion and security issues .”
For his part, José Antonio Pinilla, CEO of Asseco Spain , states that “as AI models continue to advance and their use increases, the challenge will be to maintain sufficient computing capacity to support them.” In fact, he reports that “there is already talk that the lack of computing power is an obstacle to the development of AI.” “The key will be to turn to supercomputers , to new hardware architectures or, directly, to invest in cloud computing infrastructure and data centers ”, he adds.
Industry response
The rise of AI poses many challenges, but the industry is already responding. “The AI boom has certainly led to a significant increase in the needs for computing power and data transmission. To meet these needs, the technology industry has been working on the improvement and development of new technologies, infrastructures and services”, says Vadillo.
“In terms of computing power, new graphics processing units (GPUs) and tensor processing units (TPUs) have been developed and commercialized that are specially designed for the acceleration of deep learning and other AI applications. In addition, cloud computing services have been developed and improved , which allow companies and users to access scalable and flexible computing resources, without the need to invest in their own infrastructure”, he specifies.
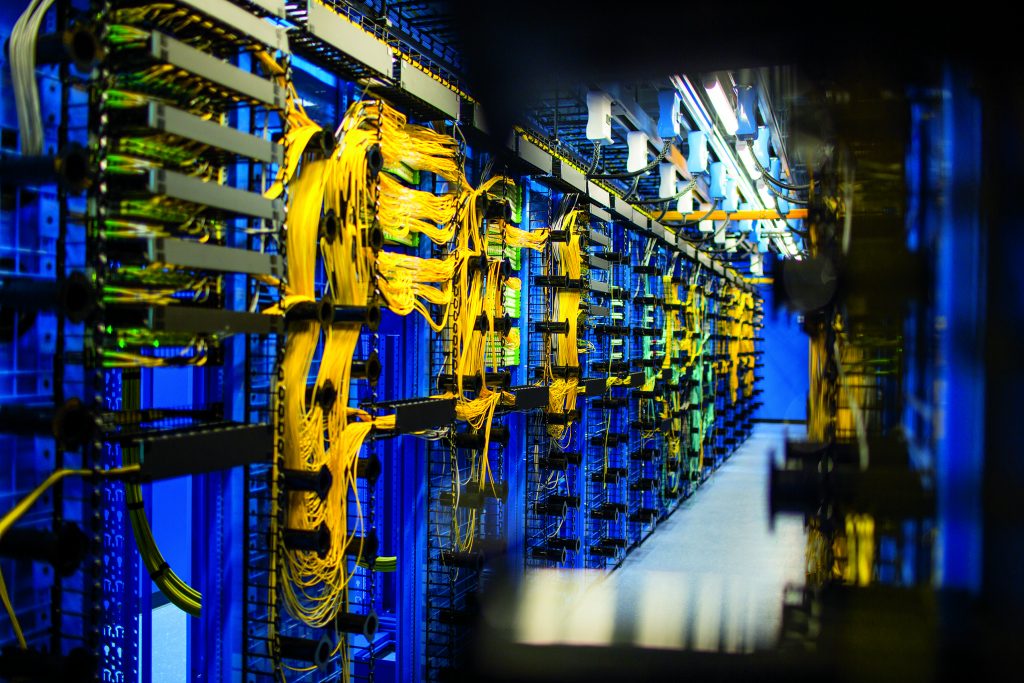
Regarding data transmission, he points out that “telecommunications companies have been improving and expanding their broadband networks and have been investing in new technologies, such as 5G, to offer faster transmission speeds and responsiveness.”
In addition, remember that “new technologies are being developed, such as fog computing, which allows data to be processed at the periphery of the network, which reduces the amount of data that must be transmitted through the central network ”.
Exponential growth of needs
The demands that the development of AI entails are very great. “Regarding computing needs , OpenAI already estimated in 2018 an exponential growth in resources for training large models, doubling resources every 3 or 4 months , and not every two years as we were used to by the ‘Law of Moore’. Consequently, we find ourselves in a stressed market, which needs to continue training models with a high consumption of resources and that demand more power in less time”, specifies the OVHcloud product marketing manager.
Also read : ChatGPT will boost spending on chatbots in the retail sector
Regarding data traffic , Sosa cites a Cisco study, which states that “it could quadruple in the next five years.” “While it is true that it is partly due to the development of the IoT and the increase in devices connected to the internet, also the growth of data generated by and for AI models could have a considerable impact on traffic, possibly causing an increase in technology investments and network deployments worldwide”, he points out.
Likewise, the head of Akamai points out that “some studies suggest that the demand for computing capacity and data transmission for AI applications could increase significantly in the coming years.” “According to a report by the McKinsey Global Institute, data traffic is expected to increase by 45% annually through 2025, driven in large part by the growing adoption of AI. Furthermore, the demand for computing capacity for AI applications is expected to increase at a CAGR of 25-30% over the next five years .”
In addition, Pinilla stresses that “hardware is representing a bottleneck for the development of AI and for meeting the need for computing and data traffic.”“Traditional computer chips, or central processing units (CPUs), are not well optimized for AI workloads. This results in reduced performance and increased power consumption. For example, the GPT-3 model, one of the largest ever created, has 175 billion parameters, and according to research by Nvidia and Microsoft Research, even if they are able to fit the model on a single GPU, the high number of computational operations required can lead to excessively long training times. So the GPT-3 is estimated to take 288 years on a single Nvidia V100 GPU,” he details.
In any case, Vadillo warns that “these estimates may vary depending on factors such as the rate of adoption of AI, the energy efficiency of computing technology, and the availability of scalable computing resources.”
Environmental impact
The increase in the requirements necessary for the development of AI can also have repercussions from an environmental point of view.
“If not designed efficiently by enterprises, AI models can consume a lot of energy , having to process massive volumes of data or run numerous iterations on the data to ensure accuracy and statistical significance in model results,” he says. the head of Equinix.
According to Bloomberg , training an AI model can consume more electricity than 100 homes. For example, training GPT-3 required 1,287 gigawatt-hours, according to research published in 2021 . This is almost as much electricity as 120 homes consume, since one home uses approximately 10 gigawatt-hours.
“According to the International Energy Agency, it is estimated that, by 2030, data centers alone will consume 1,200 terawatt hours of electricity per year , which is equivalent to the total electricity consumption of Japan and Germany combined ”, points out the CEO of Asseco Spain.
Similarly, Vadillo points out that “it is estimated that energy consumption by data centers around the world could increase by 30% in the next decade”, with the greenhouse gas emissions that this entails. “Power generation is one of the main sources of greenhouse gas emissions, so increased power consumption by AI systems could increase CO 2 emissions . According to some studies, the CO 2 emissions associated with the use of AI could reach 4 gigatons by the year 2030”, he reports.
Likewise, Pinilla notes that “a study from the University of Massachusetts indicates that the carbon footprint of training an AI model is equivalent to the emissions during the useful life of five cars . “
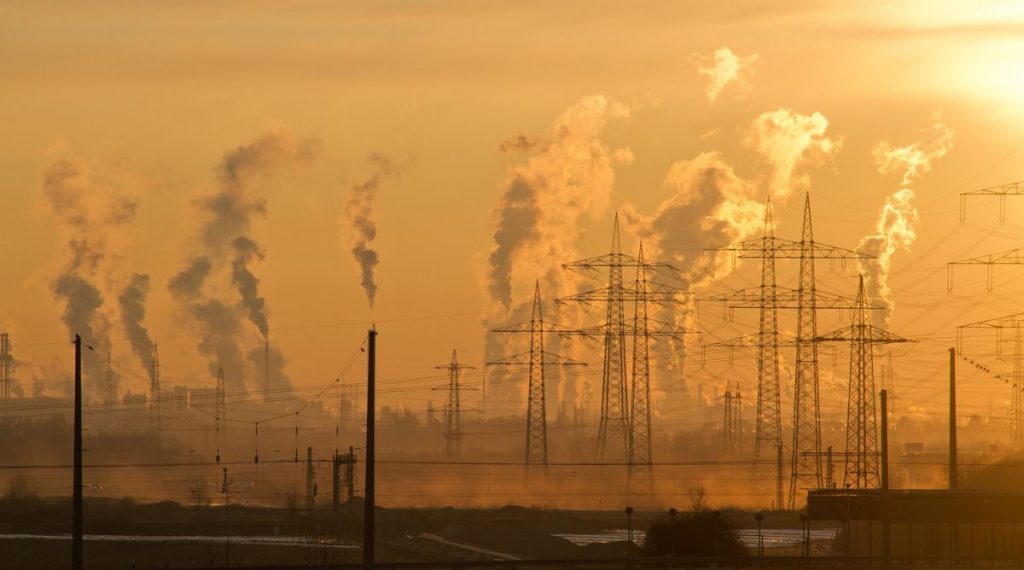
The Akamai expert also talks about other unwanted consequences, such as increased water consumption. “Data centers need large amounts of water for cooling, which can have a significant impact on local water resources. Additionally, water scarcity may limit the location of data centers in some geographic areas.”
In addition, the extraction of raw materials can pose another problem. “The production of electronic equipment and other components for AI systems requires the extraction of raw materials such as metals and minerals, which can have a significant impact on the environment,” says Vadillo.
Also read: 10 ways in which artificial intelligence benefits the Human Resources department
Finally, he considers that the rise of AI could affect the growth of electronic waste. “The rapid obsolescence of electronic equipment and the need to constantly update AI systems can create large amounts of electronic waste, which can be difficult to manage and can have a significant impact on the environment.”
Take a responsible approach
The technology industry is aware of these challenges and knows that it has to act accordingly. “It is very important to think about the sustainability strategy and adopt a responsible approach to AI,” says the head of Equinix.
Likewise, Clarisa Martínez, director of the Capgemini Spain Center of Excellence for data, analytics and AI, stresses that “it is very important to measure and monitor , with faster and more precise data, on the carbon footprint and the impacts on sustainability; calculate the carbon footprint of AI models; develop efficient machine learning architectures and optimized systems for training ; and increase transparency , including emission measurements, along with performance and precision metrics.”
In addition, the head of Akamai indicates that we must focus on “promoting energy efficiency, the use of cleaner technologies, recycling and proper waste management, research and development, and the implementation of more sustainable policies and regulations.
Thus, he points out that “measures can be applied to improve the energy efficiency of data centers and of the AI systems themselves , such as the use of renewable energy and the improvement of equipment design and cooling .” Likewise, Cano points out that data centers “have to maximize their efficiency in terms of PUE (Power Usage Effectiveness), water consumption and other elements” to face the AI boom.
In the same way, Pinilla emphasizes the use of “the use of renewable energies such as wind or solar, the use of reused water and the improvement of cooling processes and hardware from an energy point of view” as measures to increase energy efficiency and reduce greenhouse gas emissions.

Vadillo also points out that ” cleaner technologies can be used in the production of electronic equipment , reducing the extraction of raw materials and using more sustainable materials.”
In addition, it insists that ” recycling and proper management of electronic waste should be encouraged , through the establishment of recycling programs and the promotion of equipment reuse and repair practices.”
On the other hand, it defends that ” research and development of new AI technologies that are more efficient in energy terms and that use more sustainable materials and resources can be encouraged.”
The CEO of Asseco also talks about the need to “optimize algorithms so that data processing requires less energy”, as well as “research and develop more advanced AI technologies, which consider sustainability from the start and try to reduce their energy consumption.” and water and the generation of carbon footprint”.
Along the same lines, Cano believes that “company data science teams should strive to design AI models that are as clean and efficient as possible .” For example, he notes that “a good way to limit the carbon footprint of AI models is to identify ways to use only the necessary data that is most relevant, without compromising model quality.”
Finally, the Akamai expert emphasizes that “politicians can establish policies and regulations that encourage the adoption of more sustainable practices in the AI industry , such as incentives for the adoption of renewable energies, the implementation of regulations for the proper management of electronic waste and the promotion of more sustainable practices in the production and design of electronic equipment”.